AI technologies are used in virtually every aspect of our daily lives. Teachers use AI-powered tools to automate grading, while chatbots assist customers with inquiries.
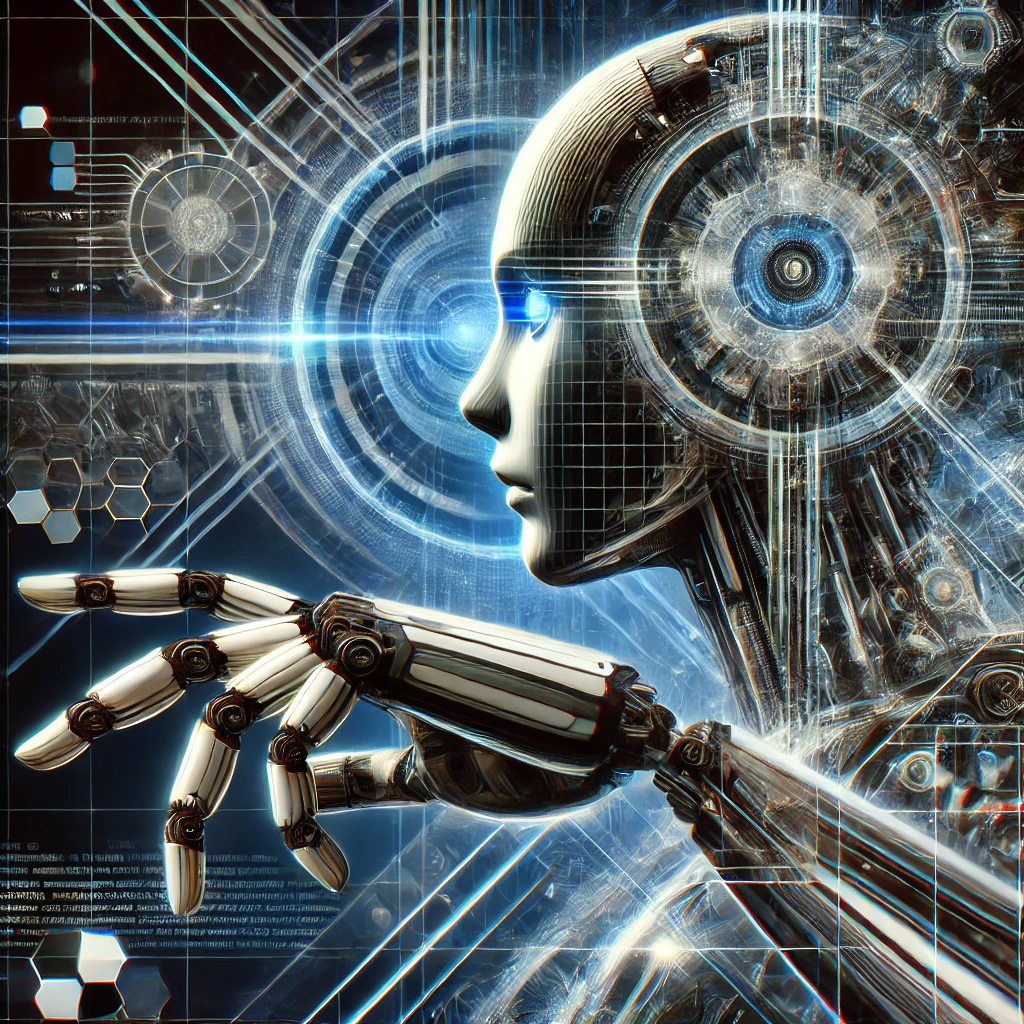
AI-powered software assists developers in writing, testing and debugging programs quickly while simultaneously helping identify and fix bugs in existing systems rapidly. AI tools are also revolutionizing healthcare delivery by automating diagnostic procedures and providing access to healthcare experts from remote locations.
AlphaDev
After the success of the AlphaZero AI system, which learned board games at an impressively superior level, DeepMind researchers took this concept a step further by building AlphaDev, an artificial intelligence that writes code itself – an incredible advancement in artificial intelligence technology. AlphaDev uses reinforcement learning techniques to develop computer algorithms that are superior to those written by human programmers.
To achieve this goal, the team designed single-player coding challenges as single-player games that could be won with artificial intelligence (AI). They employed this strategy when searching for more efficient algorithms for introductory computer science functions like sorting; their efforts yielded improved sorting algorithms that are up to 30% faster than current benchmarks.
AlphaDev algorithms are already being utilized by developers all around the world to accelerate apps and programs, but that is only just the start. DeepMind believes the approach can find many more small improvements that can then be fed back into higher-level coding languages and quickly distributed through open sourcing.
Potential advantages are manifold; drug discovery innovation could accelerate significantly with AI systems that directly predict binding affinity between a candidate drug and its target protein, helping shorten commercialization timelines by years or decades.
GNoME
Google DeepMind’s artificial intelligence system, GNoME (Graph Networks for Materials Exploration), can accurately predict the structures and stabilities of millions of new materials. It aims to speed up the discovery of high-tech materials needed for sustainable energy, computing, and other industries.
Scientists traditionally have discovered new materials through an exhaustive process of trial-and-error experimentation that often takes months or years and only yields limited results. AI-guided algorithms like GNoME may aid this process but have their limits when it comes to reliably predicting crystal structures.
Gnome has successfully overcome this hurdle by training its model on massive datasets and employing an active learning loop that continuously updates predictive models based on real-world validation, enabling it to evaluate and select promising candidates for experimental synthesis quickly. GNoME found 2.2 million potential inorganic crystals, of which 380,000 are predicted as the most stable and will serve as candidate materials for lab synthesis moving forward – this represents an eightfold increase in knowledge regarding most stable inorganic crystals. Furthermore, its findings included 52,000 two-dimensional materials similar to graphene as well as 25 times more lithium-manganese oxide compounds that may improve battery performance for electric vehicles.
GNoME marks another step forward for generative AI. This area has drawn considerable interest among both researchers and business leaders due to its ability to solve complex scientific problems and advance human knowledge. Furthermore, this technology can also be utilized to create products such as augmented reality or autonomous vehicles. As reported by PYMNTS previously, this industry will likely experience rapid expansion over the coming decade with an abundance of customized tools tailored for specific uses.
ChatGPT
ChatGPT’s debut in November 2022 marked a significant step forward for AI technology. The language model could engage in human-like conversations without prompting, prompting immediate feelings of excitement as well as fear among businesses that it could replace them altogether.
GPT (Generative Pre-trained Transformer) is an AI model trained using both reinforcement learning and supervised learning techniques. It uses a large vocabulary of human phrases for analysis before simulating human responses with its knowledge of communication patterns similar to theirs.
GPT will soon become capable of understanding context and producing more pertinent content, making it an even more versatile tool that can assist with creative writing, technical problem-solving and even language translation tasks. Furthermore, its AI capabilities will allow it to respond appropriately when asked follow-up questions arise, admit fault or refuse inappropriate requests.
ChatGPT stands out from other bots by engaging in human-like dialogue, recalling previous answers, and adapting its responses based on experience. This aspect of machine learning enables it to provide more natural, user-friendly responses.
Fear-mongering aside, AI technology advancement should not lead to fearful predictions that humans will soon become obsolete and replaced by machines. ChatGPT and similar AI tools make life more efficient for business purposes – not something to fear but instead welcomed as tools to reach our goals and enhance lives.
MedQA
Quality annotation of medical images and videos is vital in diagnosing diseases or aiding surgeons during surgeries, yet manually annotating high-resolution images at scale is very difficult. AI provides a solution by automating this process by creating question-answer data sets from raw medical images, videos, and documents – freeing physicians up to focus on patient experience while driving breakthroughs in healthcare innovation.
An AI-enabled quality assurance (QA) system could, for example, detect nerves, muscles or organs in surgical videos to improve robotic surgeon techniques and ensure positive post-op health outcomes for their patients. Furthermore, large datasets containing medical records, staffing patterns or equipment utilization information could be analyzed in order to optimize hospital operations and maximize patient health outcomes.
AI technology also plays an invaluable role in medical research when identifying and predicting drug targets, which is often difficult without access to a large set of sample data points. BioXcelerate (a division of Optima Partners Limited) has created PleioGraph as an advanced genomic AI tool that is capable of scanning large medical datasets and detecting biological networks related to disease risk, helping speed up drug discovery processes.
Other notable AI advancements this year include an FDA-cleared AI system that uses deep learning to diagnose retinal diseases using eye scan data from patients and an innovative soft robot reminiscent of Venus flytrap’s intelligent prey capture mechanism to capture insects into its artificial “mouth.” A team of researchers is also currently developing an AI platform capable of identifying optimal coral restoration sites by analyzing oceanographic data such as temperature and acidity levels as well as existing marine life.
BLOOM
BLOOM is a deep learning model capable of producing new images, text, audio, and synthetic data without human intervention. Trained on large datasets that it learns to interpret and manipulate, BLOOM represents one of the year’s most significant advances in AI technology – creating high-quality content without human interference and creating quality output without replacement or enhancement from humans alone. This marks an incredible leap forward for AI as computers now do something only humans are incapable of doing themselves!
AI can fuel growth through two channels. One way it does this is by increasing the productivity of existing products of firms using it, either through replacing human labour with AI to reduce operating costs or improving operational efficiency with better forecasting of inputs (Agrawal et al., 2019; Acemoglu & Restrepo, 2021). Empirically, however, investments in AI do not appear to increase sales per worker or total factor productivity (Ganglmair et al., 2021).
AI models are also being deployed to speed up scientific progress. Reinforcement learning models such as BLOOM help scientists improve hydrogen fusion reactions, optimize energy usage and generate new antibodies – with increasing emphasis being put on AI in research and development in the future.
Recent spikes in federal AI spending, particularly by DoD, mark a transition from experimentation to deployment of AI technologies. Both the rapid increase can see this trend in both funding obligation and the potential value of contracts awarded, although its exact causes remain unclear. Defence applications were likely chosen due to increased competition or security threats as motivations behind their investments.